Scheduling Jupyter notebooks
Scheduling Jupyter notebooks means running them automatically at specified times. This allows you or your team to rely on the results of a notebook without having to manually run it. For example, you could keep a dataset up to date by periodically pulling from a data source. Or you could run a notebook that generates a report and send it to your team over email or Slack.
Jupyter has no mechanism for scheduling notebooks. You can use additional tooling to do this for you, or you could use a Jupyter-compatible tool that has scheduling built-in.
Scheduling Jupyter notebooks locally
To schedule a Jupyter notebook, you’ll need to write a script that runs on the schedule you want. This is usually done using cron to schedule, and something like nbconvert or papermill to execute the notebook.
For example, this blog post shows how to use papermill and cron to schedule running a notebook.
The risk with this is that the computer needs to always be running. If you run this script on your local computer, for example, every time you turn the computer off or log out the schedule will stop running. You’ll need to run this script in the cloud to get it running reliably.
Use a Jupyter-compatible notebook with scheduling built-in
It’s much easier, and much more reliable, to use a tool with scheduling built-in. These tools let you write Jupyter notebooks like you’re familiar with, and have an easy-to-use UI for creating schedules to run notebooks on. Most of them also notify you if something goes wrong.
Below are some notebook tools that are Jupyter-compatible and have first class support for scheduling.
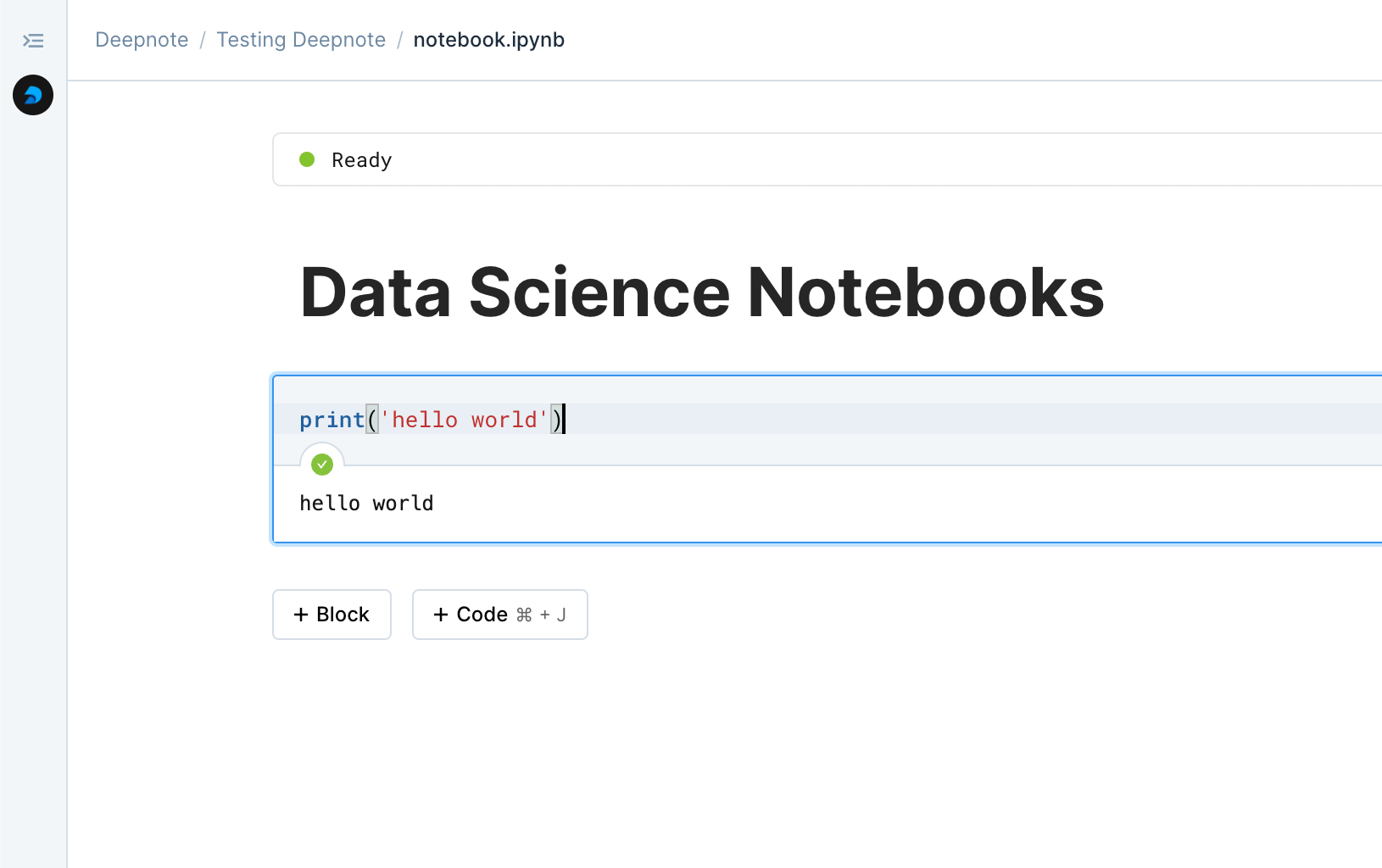
Deepnote
Deepnote is a new kind of data notebook that’s built for collaboration — Jupyter compatible, works magically in the cloud, and sharing is as easy as sending a link.
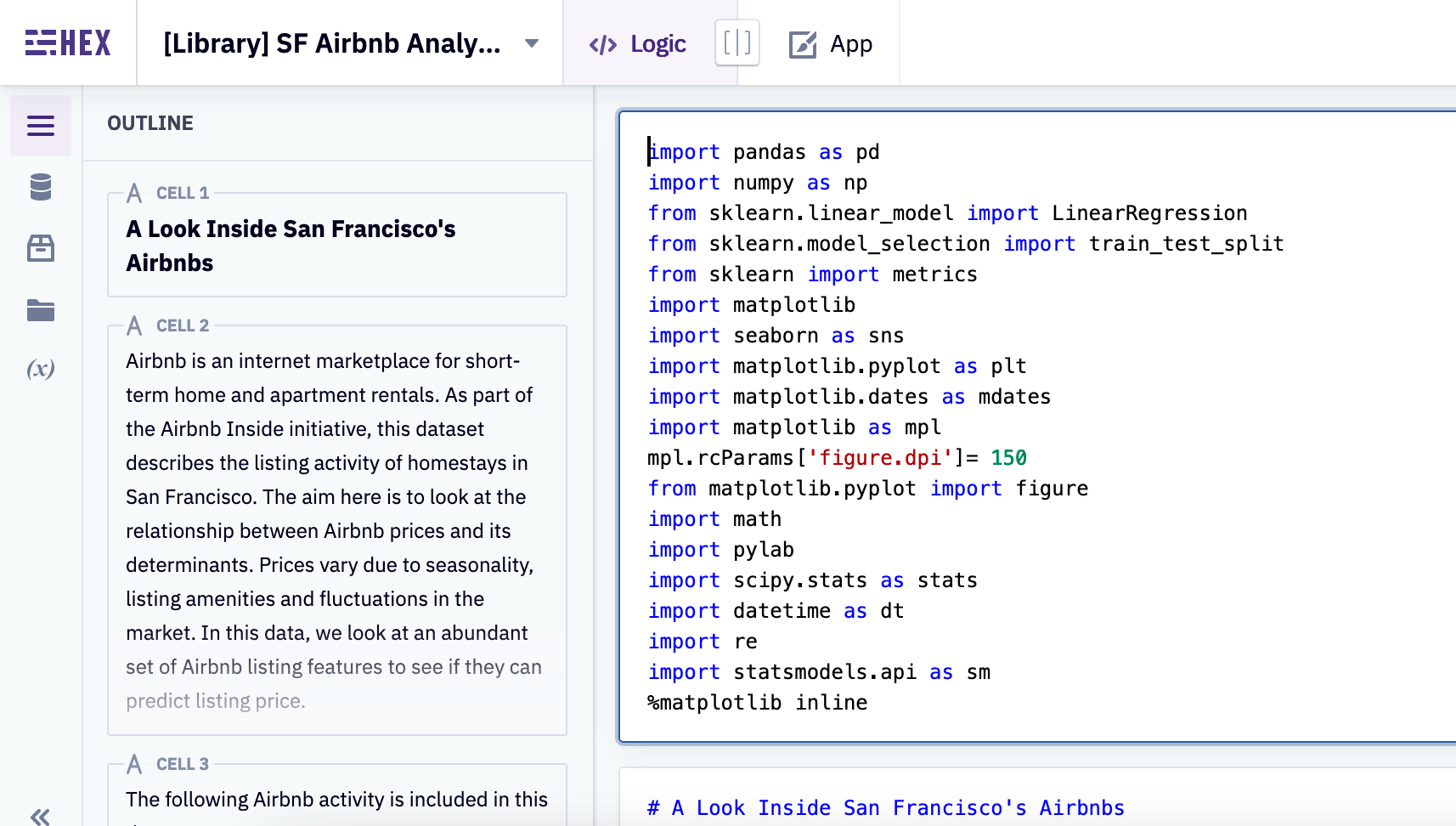
Hex
The Data Workspace for Teams. Work with data in collaborative SQL and Python notebooks. Share as interactive data apps that anyone can use.
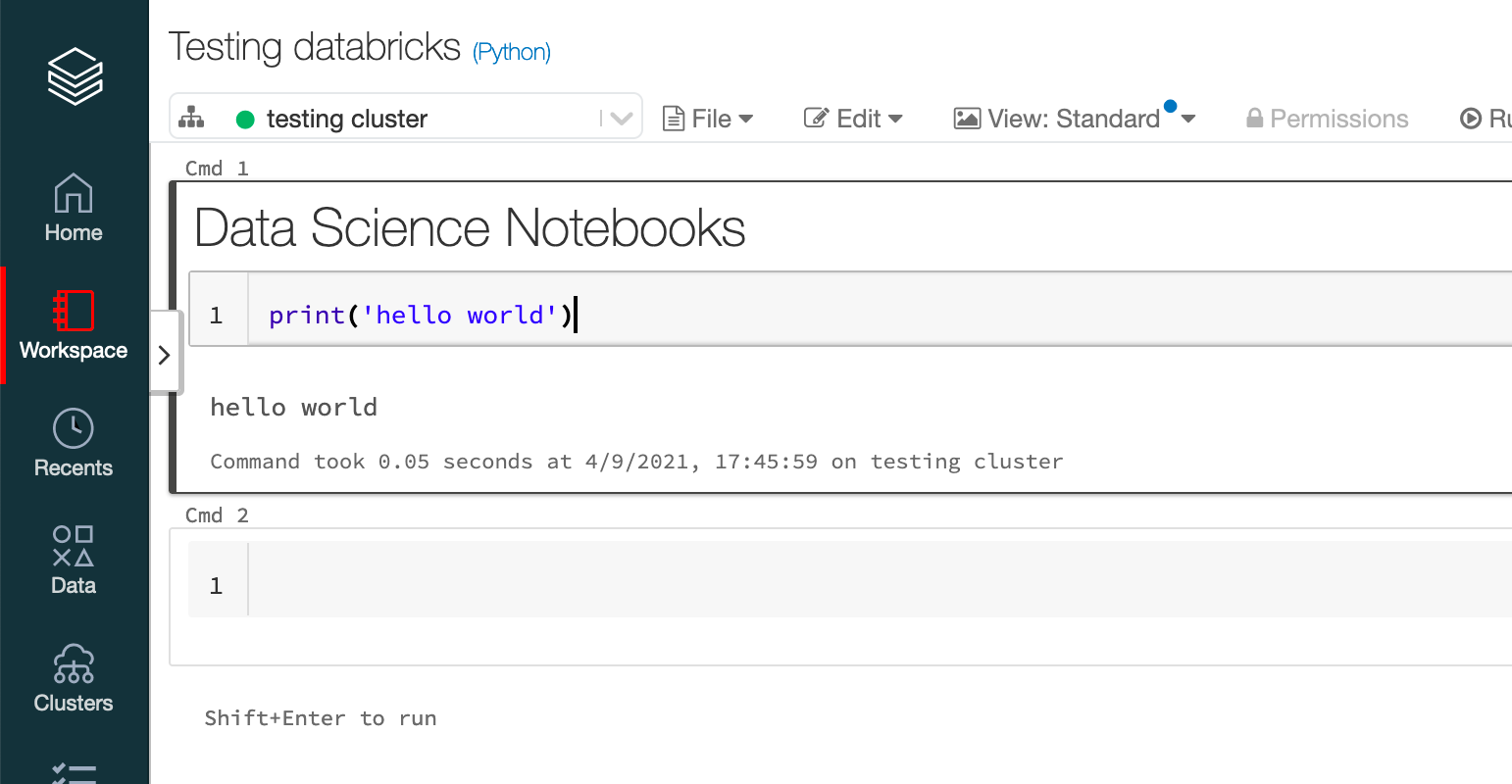
Databricks Notebooks
Collaborate across engineering, data science, and machine learning teams with support for multiple languages, built-in data visualizations, automatic versioning, and operationalization with jobs.
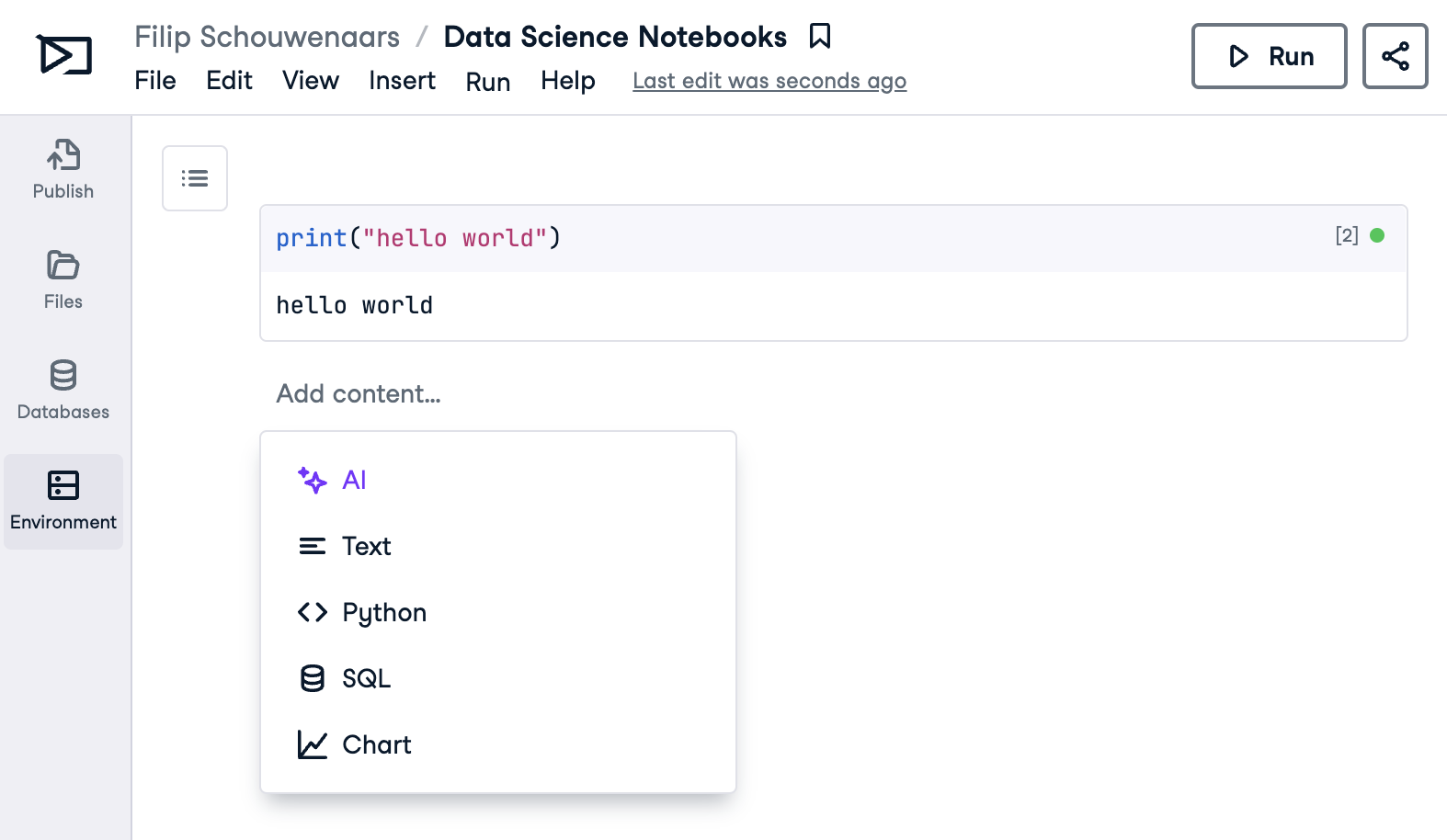
DataCamp Workspace
DataCamp Workspace is an AI-powered data notebook to help you get from data to insights, faster.
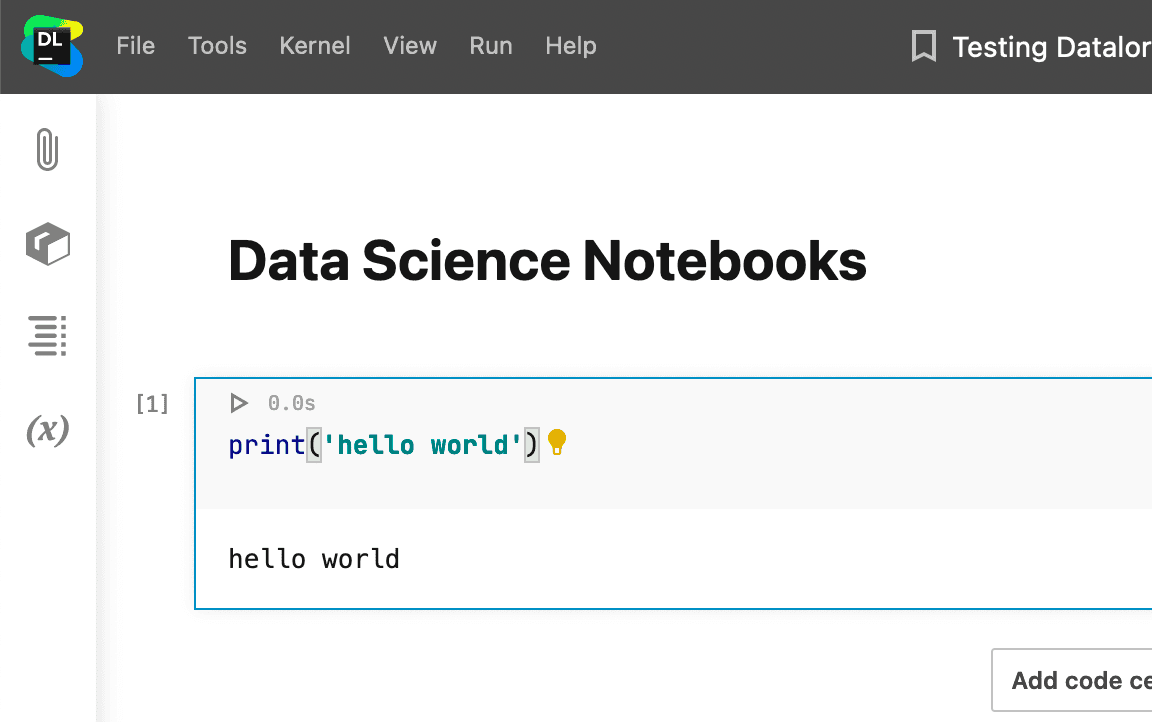
Jetbrains Datalore
A powerful online environment for Jupyter notebooks. Use smart coding assistance for Python in online Jupyter notebooks, run code on powerful CPUs and GPUs, collaborate in real-time, and easily share the results.
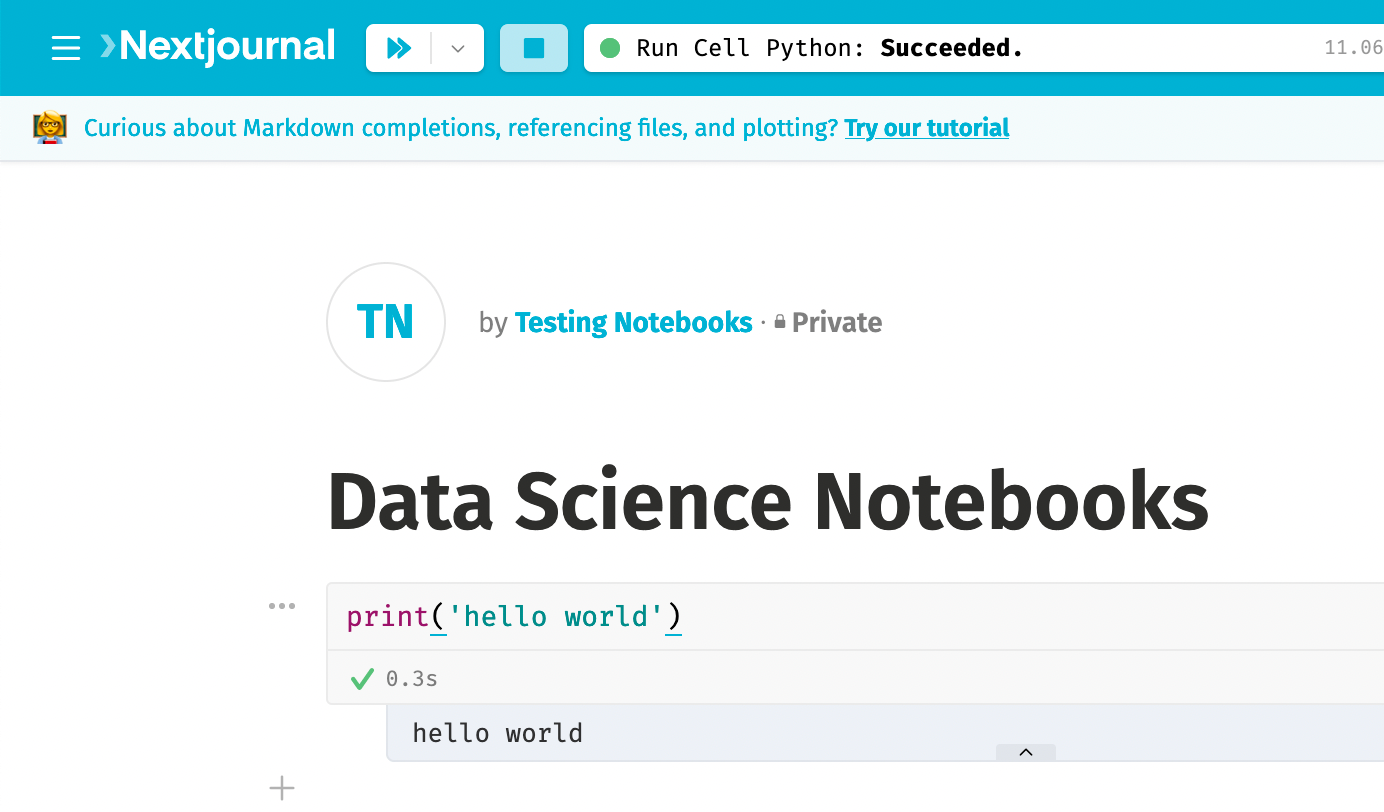
Nextjournal
Runs anything you can put into a Docker container. Improve your workflow with polyglot notebooks, automatic versioning and real-time collaboration. Save time and money with on-demand provisioning, including GPU support.
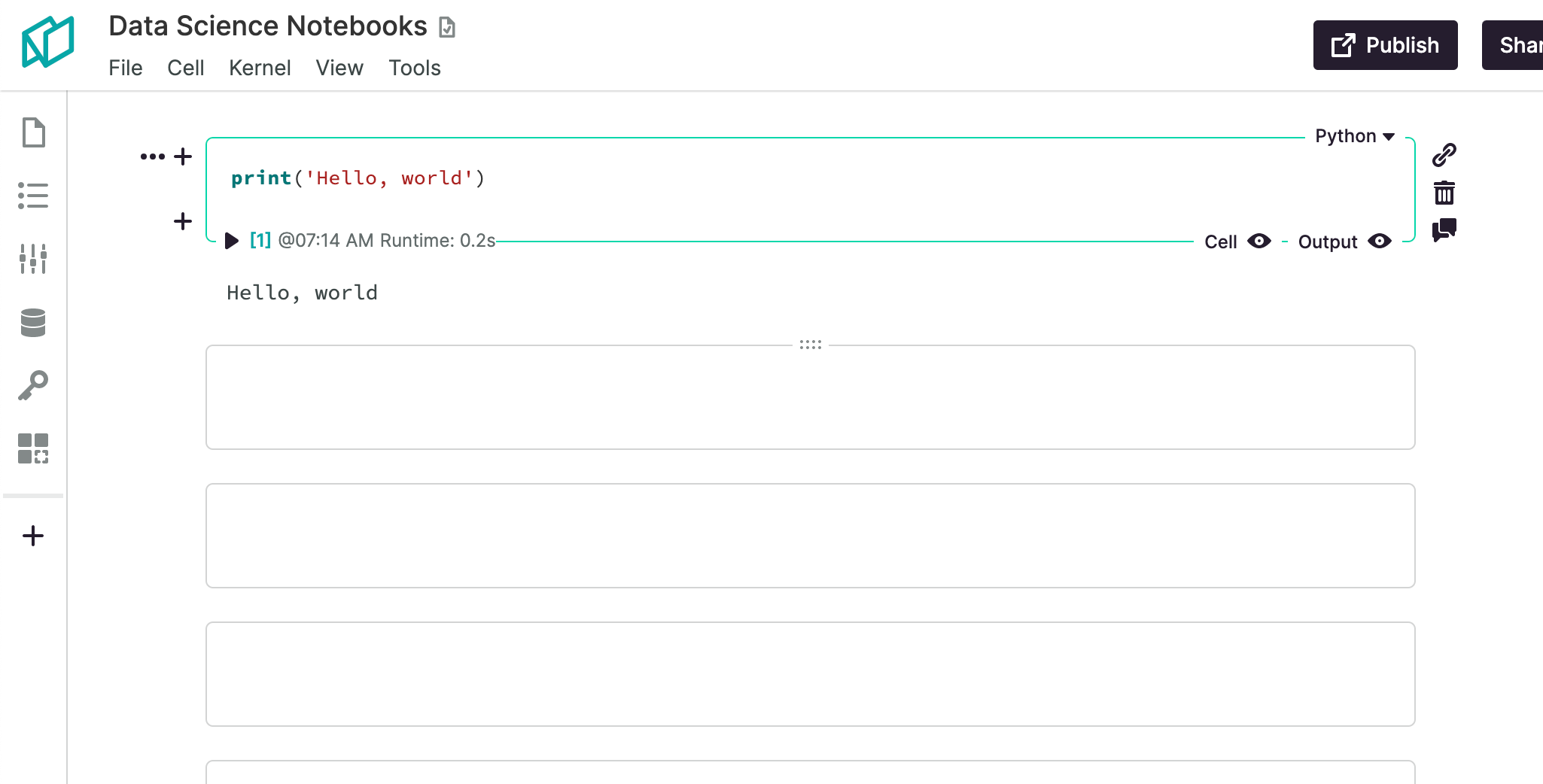
Noteable
Noteable is a collaborative notebook platform that enables teams to use and visualize data, together.